I just attended the digital methods summer school, hosted by University of Amsterdam initiative of the same name. It’s something I’ve wanted to do for years, but first had the opportunity as a phd candidate. It was worth the wait, and here’s a quick summary of what I learned about the methods, the tools, and the course.
The methods
“Digital methods” could mean a lot of different things, but there’s a lot at stake in the rhetoric. Digital humanities, data journalism, webometrics, virtual methods, data science, oh my. Cramming the internet into social science research makes for a complicated landscape, and there’s ontological and political work to be done in how academic schools and approaches distinguish themselves.
Digital methods stakes out its turf with a 2-part move:
- using digitally native methods (methods which are qualitatively different than what scholars were capable of 20 years ago, ie: not just digitization of familiar methods for increased efficiency)
- to analyze digitally native phenomenon (ie: things that only take place in a digital information ecology).
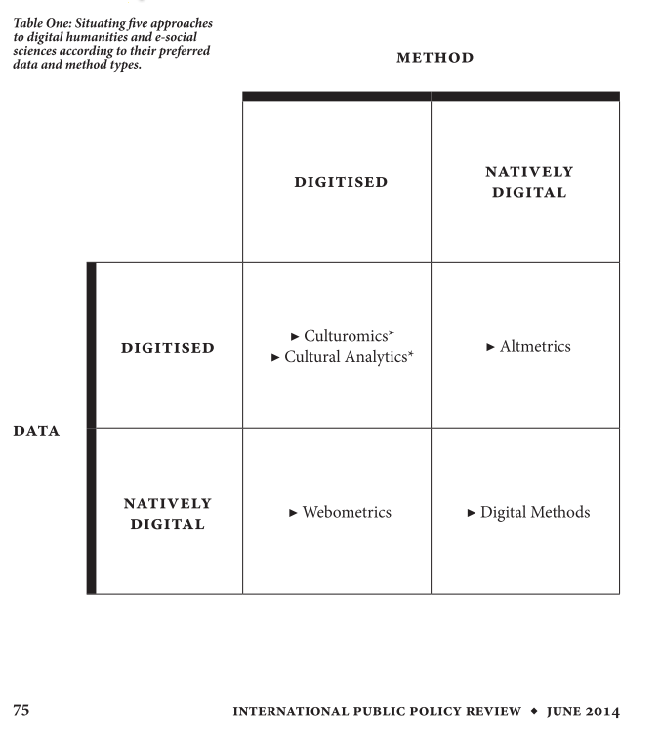
These are very deliberate distinctions, and they have some interesting consequences.
digitally native methods
The first tenet places an emphasis on a technical savvy that you don’t find many places in the academy. For younger academics without hard engineering skills, this can lead to frustration when using complicated tools just beyond their capacity, or significant time spent learning tools or scripts. It also opens interesting opportunities for collaborations with data scientist and NGO techies, but with methodological pitfalls.
https://media3.giphy.com/media/5f5o0WiJVXFfy/200.gif
Relying on others to do your data crunching can be the methodological equivalent of having other people pack your parachute. Data scientists, especially those who do a lot of work with civil society, tend to be interested in outcomes – whether insights or simply code that works. It’s difficult for them to spot methodological nuances about representivity or how data reflects empirical dynamics, but they do tend to work in authoritatively and within a black box. This can in turn lead to hidden methodological shortcuts when a team has mutually exclusive technical skills. In one of our summer school projects this was as mundane is miscommunication about who qualified as a “sender” on an email list.
This type of thing is manageable, of course, but using digitally native tools with which a researcher who isn’t infinitely familiar with them can mean that some methodological steps take place out of site. Ironically, this also means that the chances of having methodological shortcuts called out by other researchers also decrease. So, cause for caution.
digitally native objects
The second tenet raises interesting questions about what studying digitally native data can actually tell us about the empirical world. What do tweet sets actually tell us about online conversation or conversation in general? When and how does text on a website represent an organization’s position on an issue? Can Github contributions be considered proxies for the collaborative dynamics of developer communities?
These questions are especially challenging given how private companies tend to limit researchers’ data access (be it 1% rate limits or exclusion of key social data), but at bottom it means that digital methods imply specific qualifications about the insights they produce. Those qualifications can be easy to forget when reasoning through an analysis (especially when flashy digital tools actually work and show you a surprising relationship), and even easier to miss when reading research conclusions (especially when flashy visualizations show you a surprising relationship). At bottom, this might mean that it’s particularly incumbent on researchers using digital methods to continuously ask themselves about what data is missing from their analysis, and make those considerations explicit in their results.
I was also struck by the feeling that digital methods remain remain quite young. This both in the sense that they are practiced and described by a fairly small group of researchers, and that there is a significant amount of approximation involved in any methodological design. I get the sense that these methods are powerful and exciting, but choose to adopt them and you can expect to shrug your shoulders occasionally as you guess your way towards the best approach.
The literature on the methods also seems to lag a bit behind what is already being done by data scientists and civic hackers on the same kinds of data. A paper on heuristics for reading network visualizations, for example, codifies distinctly basic tenets of common gephi interpretation, and the paper on designing research queries offers a method that seems commonsensical. Of course, this is because that’s how science works. The academy doesn’t plunge ahead, it methodically asserts and contests ideas on an iterative basis, slowly establishing and building on tested conclusions.
But there is quite a bit of research written up on digital methods, and while the practical methodologies themselves would be familiar to anyone who has been to a data expedition or two, the theoretical justification is important, and the breadth of their application was both inspiring and surprising. I’ve pasted links to articles in the summer school reader at the bottom of this post, which gives a good feeling for this literature.
For those who want more practical guidance on different methodological approaches, the DMI wiki has research protocols that are as wide ranging as they are perfunctory and pragmatic.
The course
I showed up at the summer school not knowing what to expect, and was surprised by the format. The school ran over two weeks, each of which proceeded with:
- 1 day of academic lectures spanning a variety of issues (plus some “tutorials” in the first week, though these felt more like lectures)
- 4 days of project workshopping in small groups, following a workshop method reminiscent of a data expedition, and concluding with a presentation to the entire summer school.
The projects in each week were led by either visiting scholars or local activists and phd candidates. Other participants were permitted (if not encouraged) to pitch their own projects in the second week.
I was expecting a much more academically styled working school, but was familiar enough with the workshop method to find this comfortable. I worked on a project evaluating the online connections and discussions surrounding digital rights advocacy in Europe (using Twitter data) and a project examining how civil society actors successfully lobbied for the inclusion of human rights in ICANN policy (using public mailing list data).
Based on this, and several conversations with participants in other groups, here’s my advice to future summer/winter school participants on choosing groups.
- The group facilitator matters. Beware projects that aren’t clearly formulated because lack of clear mandate and a heterogenous group can lead to a lot of frustration and sitting on your hands.
- Tools matter. This might be obvious, but substantive insights over the course of the week are limited, choose projects based on their use of tools you want to learn how to use.
- Ambition matters. Projects dont seem to manage anything they hope to, expect to only address the first half of what they propose, especially if they propose multiple tools or data sets.
- Group size matters. Large groups will produce a lot of sub-groups and small analyses, many of which won’t make it into the final analyses and will get left half finished, which can be frustrating. If you’re in a large group, choose a sub group that has someone with technical or design skills, which makes those analysis more likely to survive the Darwinian process of selection in the final day.
- Data matters. Projects don’t always have the data they think they do, or all the data they think they do. If projects bring their own data, be aware of what limitations that might impose.
On a practical note:
- You won’t save much money staying at an AirBnB 20 minutes away from the venue, and what you do save you will spend on transport.
- You can rent a bike for about 100Euros if you hunt for a deal, you can buy one for about 50.
- Food is expensive, the grocery store across from the library has excellent sandwiches and salads that will cut those costs by 75%.
- I never used or referenced the readings directly in the course of the 2 weeks, and many of them are repeated in “tutorials”, but having read them immensely improved the quality of conversation I had with the people who built the tools and led the workshops.
The tools
One of the most impressive outputs of the Digital Methods Initiative is their library of tools. These are used throughout the project workshops, and developed and maintained on a running basis as far as I understand. They appear to be open for anyone to use, though of the most powerful ones require you to run them on your own server.
On the DMI wiki you will find about 70 tools for scraping popular social media, visualizing and grouping data. Each tool includes some basic instructions and documentation, but these are often not complete, and you may need to reach out or simply play around with data outputs to answer questions in the methodological black box, as described above. The people who have developed the tools seem generally very happy to answer questions, though figuring out who belongs to which might require some intensive googling, browsing of the academic literature, or simple phone calls.
The Reading List
Most of these have links to ungated versions, but the full reader is also available on the summer school wiki.
Beer, D. (2016). How should we do the history of Big Data? Big Data & Society, 3(1), 181–196. http://doi.org/10.1177/2053951716646135
Bennett, W. L., & Segerberg, A. (2012). The Logic of Connective Action. Information, Communication & Society, 15(5), 739–768. http://doi.org/10.1080/1369118X.2012.670661
Borra, E., Rieder, B., Zimmer, M., John, N., Bruns, A., & Weller, K. (2014). Programmed method: developing a toolset for capturing and analyzing tweets. Aslib Journal of Information Management, 66(3), 262–278. http://doi.org/10.1108/AJIM-09-2013-0094
Couldry, N., & Powell, A. (2014). Big Data from the bottom up. Big Data & Society, 1(2), 2053951714539277. http://doi.org/10.1177/2053951714539277
Diminescu, D. (2012). Introduction: Digital Methods for the Exploration, Analysis and Mapping of e-Diasporas. Social Science Information, 51(4), 451–458. http://doi.org/10.1177/0539018412456918
KOK, S., & ROGERS, R. (2016). Rethinking migration in the digital age: transglocalization and the Somali diaspora. Global Networks, (Kurz 2012), 1–24. http://doi.org/10.1111/glob.12127
Madianou, M., & Miller, D. (n.d.). Introduction. In Migration and New Media: Transnational Families and Polymedia.
Powell, A. (2016). Hacking in the public interest: Authority, legitimacy, means, and ends. New Media & Society, 18(4), 600–616. http://doi.org/10.1177/1461444816629470
Renzi, A., & Langlois, G. (2013). Data Activism.
Rieder, B. (2013). Studying Facebook via Data Extraction: The Netvizz Application. Proceedings of WebSci ’13, the 5th Annual ACM Web Science Conference, 346–355. http://doi.org/10.1145/2464464.2464475
Rogers, R. (2015). Digital Methods for Cross-platform Analysis : Studying Co-linked , Inter-liked and Cross-hashtagged Content. In ???????????????????????? ????? (pp. 1–24).
Rogers, R. (2014). Political Research in the Digital Age. International Public Policy Review, 8(1), 73–88. http://doi.org/10.1177/1745691612459060.
Rogers, R. (2016). Foundations of Digital Methods: Query Design. In The Datafied Society. Amsterdam: Amsterdam University Press. http://doi.org/10.1017/CBO9781107415324.004
Schrock, A. R. (2012). Civic hacking as data activism and advocacy: A history from publicity to open government data. New Media & Society, 18(4), 581–599. http://doi.org/10.1177/1461444816629469
Star, S. L. (2016). The Ethnography of Infrastructure. American Behavioural Scientist, 43(3), 377–391. http://doi.org/0803973233
van der Velden, L. (2014). The Third Party Diary: Tracking the trackers on Dutch governmental websites. NECSUS. European Journal of Media Studies, 3(1), 195–217. http://doi.org/10.5117/NECSUS2014.1.VELD
Venturini, T., Jacomy, M., & Pereira, D. (2010). Visual Network Analysis, 1–20. (ungated)